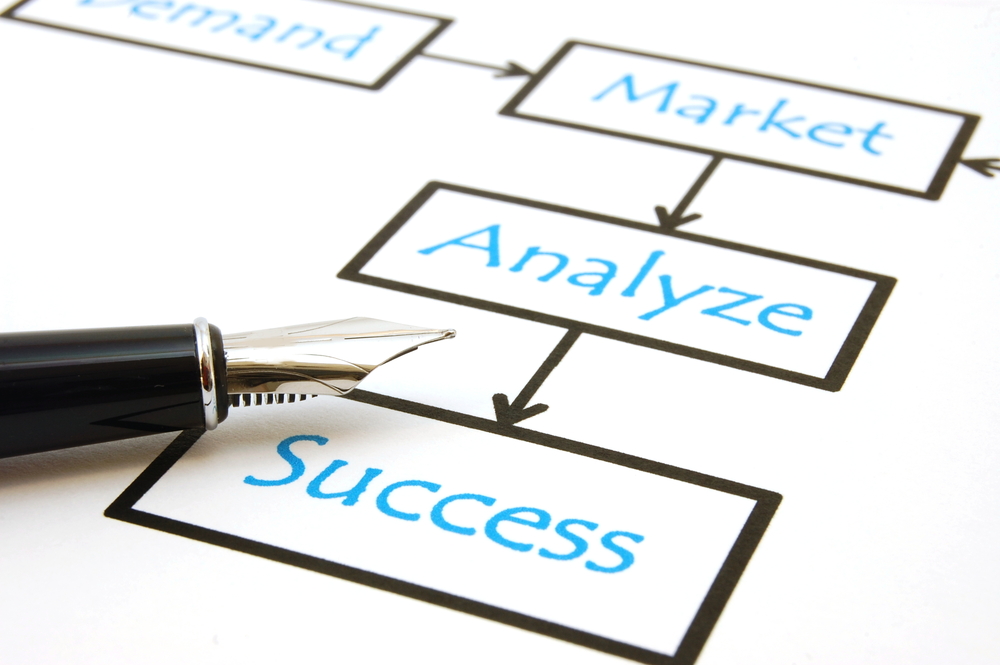
You can build data analytics to leverage your Strategic Marketing data.
‘Marketing is the management process responsible for identifying, anticipating and satisfying customer requirements profitably… Marketing as a process calls for management decisions on product, pricing, distribution, promotion and personal selling, and customer service. These elements are known as the ‘marketing mix.’
The Chartered Institute of Marketing
Data Analytic Based Strategic Marketing Solutions
The marketing management process and the marketing mix can be complex. Effective marketing planning requires not only intelligence on customers but across a wide spectrum of areas such as product lifecycle and supply chain. The reality is that strategic marketers struggle to get information from diverse parts of the business for analysis and decision making in their plans.
The power of data analytics is that they can use combined data from diverse areas of the business in an enterprise data warehouse holding data often referred to as the single version of the truth. Data in an enterprise data warehouse can be combined and turned into information.
In strategic marketing a strategic marketing plan template can be used as the core. The plan usually comprises of two key components; analysis and decision. At each relevant stage of the plan the marketer should be able to access data analytics that provide the latest data for that stage of the plan. As well as providing marketing intelligence the solution gives the ability to plan, monitor and introduce contingency if veering off track.
Visit the website of The Chartered Institute of Marketing for best practice in strategic marketing
You must be logged in to post a comment.